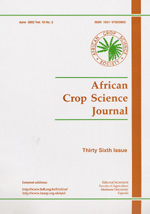
|
African Crop Science Journal
African Crop Science Society
ISSN: 1021-9730 EISSN: 2072-6589
Vol. 7, Num. 4, 1999, pp. 549-558
|
African Crop Science Journal, Vol. 7. No. 4, 1999
African Crop Science Journal, Vol. 7. No. 4, pp. 549-558, 1999
Farmers perceptions and adoption of soil
management technologies in western Kenya
M. Makokha, H. Odera, H.K. Maritim, J.R. Okalebo and D. M. Iruria
Moi University, Faculty of Agriculture, P.O. Box 1125, Eldoret, Kenya
Code Number: CS99046
ABSTRACT
A study was conducted to test two hypotheses: that farming conditions significantly
influence farmers perceptions of new agricultural technologies and probability
of adoption, and that farmers perceptions of technology-specific attributes
associated with use of new technologies significantly influence adoption decisions.
A tobit model analysis of a random sample of sixty farmers revealed farmers
participation in agricultural field days and on-farm trials to be significant
at 0.05 level in explaining adoption decisions. Other farm variables that were
significant in explaining adoption were farmers participation in agricultural
seminars and workshops (P< 0.01), contact with extension (P<0.05 level)
and decision to reduce use level for inorganic fertilisers (P< 0.01). The
social status of the farmers was not significant in explaining adoption behaviour.
Among the technology-specific attributes, reliability in supply and availability
of technologies was significant at 0.05 level in explaining adoption. The impacts
of technologies on plants growth vigour and yield were significant at
(P< 0.05). Convenience in use of technologies and labour requirements was
not significant in explaining adoption decisions. The results indicate that
early technology adopters are likely to be those who participate in local activities
that introduce and explain new approaches to soil fertility management.
Key Words: Agricultural technologies, East African Highlands, nutrient
replenishment, organic resources, tobit model
RÉSUMÉ
Une étude a été conduite pour tester deux hypothèses: que les conditions de
culture influencent significativement les perceptions des agriculteurs pour
les nouvelles tecnologies agricoles et la probabité dadoption et que les
perceptions des agriculteurs pour les caractéristiques de technologies spécifiques
associées à lutilisation des nouvelles technologies influencent significativement
les décisions dadoption. Une analyse du modèle tobit dun échantillon
aléatoire de 60 agriculteurs a révélé que leur participation dans les journées
champêtres et dans les essais en milieu réel êtait significative
à un niveau de 5% dans lexplication des décisions dadoption. Les
autres variables liés à la ferme qui étaient significatifs dans lexplication
dadoption étaient: la participation des agriculteurs dans les seminaires
et ateliers (P<0.01), contact avec vulgarisateurs 5%, et la décision de réduire
le niveau dutilisation des engrais inorganiques (P<0.001). La situation
sociale des agriculteurs na pas été significative dans lexplication
du mode dadoption. Parmi les caractéristiques de technologies spécifiques,
la fiabilité dans lapprovisionnement et la disponibilité des technologies
étaient significatives à 5% dans lexplication dadoption. Les impacts
de technologies sur la vigueur de la croissance de la plante et du rendement
étaient significatifs à 5%. La commodité dutilisation des technologies
et des exigences de la main doeuvre nétaient pas significatives
dans lexplication de la décision dadoption. Les résultats indiquent
que les adopteurs en premier des technologies sont ceux qui participent dans
les activités locales qui introduisent et expliquent les nouvelles approaches
de la gestion de la fertilité du sol.
Mots Clés: Technologies agricoles, Hautes terres de lAfrique de
lEst, restauration des nutritifs, resources organiques, modèle tobite
Introduction
A number of studies have been conducted on factors influencing
perceptions and adoption of new technologies both within and outside the sphere
of agricultural production. Current literature on adoption studies reveal three
major approaches to farm technology dissemination.
The innovation-diffusion model. This model considers access to information
about an innovation to be the key factor in determining adoption decisions.
This school of thought assumes that appropriateness of innovation is as given
and thus reduces the problem of technology adoption to communication of information
on a given technology to potential end-users. The model places emphasis on
extension contact, use of mass media and opinion leaders as a means of influencing
adoption for new technologies. Classical examples of works in this line of thought
include Rogers (1962), Agrarwal (1983), and Benor et al. (1984).
Variables which affect farmers access to information as
determinants of adoption for new technologies have also been widely used in
economic models of adoption decisions (Feder et al., 1985; Kebede et
al., 1990; Shakya and Flinn, 1991; Polson and Spencer, 1991; and Stauss
et al., 1991). However, this model has variously been criticised as being
top-down in orientation and thus lacking consideration for farm variables
in its packaging (Roling, 1988). The model has also been associated with various
problems in its implementation, particularly concerning choice of contact farmers
(Moore, 1984), poor research extension linkages (Chapman, 1988), and weak linkages
with farmers at field level (Dejene, 1989). However, quantitative assessment
of impacts of the model has revealed improvement in technology adoption and
farmers knowledge (Feder et al., 1985; Feder et al., 1986;
Feder and Slade, 1986; Hussain et al., 1994).
Economic constraint model. This model considers economic constraints
to distribution patterns of resource endowments as the major contributor to
observed adoption decisions (Aikens et al., 1975). Lack of access to
land and capital has been demonstrated as being significant constraints to
adoption decisions (Havens and Flinn, 1976; Yapa and Mayfields, 1978). Qualitative
effects of factors such as farm size, liquidity and risk attitudes on decisions
to adopt new technologies have been examined (Just and Zilberman, 1983; Harper
et al., 1990; Pitt and Sumodiningrat, 1991). Just and Zilberman (1983)
showed the impact of risk attitudes and farm size on adoption. Theoretical
work has shown that farm size affects adoption decisions through the availability
of some threshold hectarage where innovations occur. The study demonstrated
that given a series of technological components, adoption pattern is a function
of profitability, riskiness, initial capital requirement, complexity in use,
and availability of each component. Green and Ngongola (1993) documented
factors affecting fertiliser adoption in less developed countries and demonstrated
the quantitative impact of policy changes on fertiliser use among subsistence
farmers.
The adopter perception model. This model contends that farmers
subjective perceptions of new technologies in light of prevailing socio-economic
environment conditions their adoption behaviour. From the seminal works by
Kivlin and Fliegel (1966a, b, 1967), the concept of adopter perception
can now be found in varied agricultural economics literature (Norris and Batie,
1987; Nowak, 1987; Lynne et al., 1988; Gould et al., 1989;
Adesina and Zinah, 1993; Adesina and Baidu-Forson,1995). Quantitative studies
that have considered farmers perceptions in context of adoption decisions
have included farmers perceptions of a new technology. Farmers are considered
to have subjective preferences for specific characteristics inherent in new
technologies or innovations ( Ashby and Sperling, 1992). These preferences are
assumed to play a significant role in technology adoption. Adesina and Baidu-Forson
(1995) contend that adoption of technologies by farmers reflects rational decision-making
based upon farmer perceptions of appropriateness of the characteristics of the
technologies under investigation.
In this paper, the adopter perception model is employed
in analysis of farmer perceptions and adoption characteristics among subsistence
farmers in western Kenya. The paper examines variables that influence perceptions
of non-conventional soil nutrient replenishment technologies and their adoption
decisions. The paper also looks at technology-specific attributes inherent in
such technologies that condition the farmers subjective preferences.
Materials and Methods
The setting. The study was conducted in two administrative districts
within the western highlands of Kenya, namely, Siaya and Bungoma districts.
The districts are characterised by high population density, as high as 1200
persons per square kilometer (Hoekstra and Corbett, 1995), small farm holdings
with average farm size of 2.5 ha per farm-farmily; and low maize yields
ranging between 0.4 t ha-1 - 0.8 t ha-1 compared to estimated
potential of 4 t ha-1. Mean monthly per capita income is less than
the national average with high disperity between the estimated requirement
and actual purchases for inorganic fertilisers and a high proportion of farmers
who have either reduced or abandoned fertiliser use. A low extension staff
to farmer ratio exists (1:1280) with food deficits of up to 64% (Omare,
1998) and low achievement of desired extension goals (32.6%). Average net nutrient
losses of nitrogen and phosphorus are 14 and 3 kg ha-1 yr-1,
respectively, with overall widespread negative soil nutrient balances (Woomer
et al., 1997).
Due to limited use of conventional inorganic fertilisers
in the survey area, combination of organic inputs with relatively inexpensive
phosphate rock (PR) is being advocated (Reijntjes et al.,1992). Non-Governmental
Organisations (NGOs) such as International Centre for Research in Agroforestry
(ICRAF) and CARE Kenya; National Research organisations, namely the Kenya Agricultural
Research Institute (KARI) and Kenya Forestry Research Institute (KEFRI); local
NGOs such as Sustainable Community Development Programme (SCODP), Sustainable
Agricultural Centre for Research and Development (SACRED-Africa), and the
Organic Matter Management Network (OMMN); are all promoting use of non-conventional
organic resources as supplements to mineral fertilisers in an effort to replenish
the nutrient depleted soils of western Kenya (Woomer et al., 1998).
On-farm-trials conducted in the survey area using some of
the non-conventional soil nutrient replenishment technologies have shown promise.
Phosphate rock (PR) at the rate of 100 kg P ha-1 resulted in an incremental
yield response of 750 kg of maize. ICRAF using Tithonia diversifolia at
a rate of 5 t ha-1 dry matter realised 1183 kg of maize and an improved fallow
agroforestry system resulted in 2228 kg of maize ha-1 (Niang et al.,
1996).
The Phosphorus Resource Evaluation Project (PREP) is experimenting
with a technology that was specifically developed to amelioriate partches of
phosphorus deficiency, a phenomenon that characterises most of the farms in
the region. The technology (PREP-PAC) consists of 2 kg of Minjingu phosphate
rock together with rhizobium innoculant, legume seed, and urea fertiliser, which
is recommended for use on 25 m2 phosphorus deficient patches (Woomer et al.,
1998; Nekesa et al., 1999). Through a related study, Moi university is
also running on-farm trials that aim at determining farmers preferences for
phosphate rock fortified compost as a mode of applying PR. The farmers subjective
preferences are being viewed from the perspective of convenience in use, impact
on crops growth vigour, and overall yield levels associated with various technological
forms. Preliminary results from this study reveal that technology-specific attributes
associated with the mode of use of phosphate rock condition adoption decisions.
Conceptual model. Following Adesinah and Zinnah (1993), farmers
adoption decisions regarding any new technology are assumed to be based upon
utility maximisation. For the purpose of this study, we define the use of alternative
soil nutrient replenishment technologies by j, where j = 1 for a farmers
favourable decision, based on subjective perceptions regarding such technologies,
and j = 2 for a farmers favourable decision, based on subjective perceptions
regarding use of other conventional inorganic fertiliser sources. The non-observable
underlying utility function, which ranks the preference of the ith farmer is
given by U(MJI, AJI). Thus, the utility derivable from
the form of soil nutrient replenishment strategy depends on M which is a vector
of farmer-specific attributes of the adopter and, A which is a vector of technology-specific
attributes. Although the utility function is unobserved, the relation between
the utility derivable from a jth technology is postulated to be a function of
the vector of observed farmer-specific characteristics such as social standing
in society, participation in field-days, agriculture training workshops, and
on-farm trials and contact with extension agents. Technology-specific characteristics
include the impact of the technology on yield, availability of the technology
on the farmers farm or in the immediate neighbourhood, convenience in
use, labour requirement and impact of the technologies on crop growth vigour
in early stages of plant growth. Expressed mathematically, this is represented
as:
Uji = ejFi(Mi, Ai) +
eji j = 1,2,; i = 1,....,n [Equation 1]
Equation 1 does not restrict the function F to be linear.
As the utilities Uji are random, the ith farmer will select the alternative
j = 1 if U1i>U2i or if the non-observable (latent) random variable y* =
U1i - U2i > 0. The probability that Yi equals one (i.e., that the farmer
adopts use of alternative soil nutrient strategy) is a function of the independent
variables, expressed mathematically as follows:
Pi = Pr (Yi = 1) = Pr(U1i>U2i)
= Pr[e1Fi(Mi, Ai) + e1i >e2Fi(Mi, Ai) + e2i ]
= Pr[ e1i - e2i > Fi(Mi, Ai) (e2 - e1 ) ] = Pr(ei> - Fi (Mi, Ai)e)
= Fi(Xie)
[Equation 2]
where X is the n x k matrix of the explanatory variables,
and e is a k x 1 vector of parameters to be estimated, Pr(e) is a probability
function, ei is a random error term, and Fi(Xie) is the cumulative distribution
function for ei evaluated at Xie. The probability that a farmer will adopt the
use of alternative soil nutrient replenishment techno-logies is a function of
the vector of explanatory variables and of unknown parameters and error term.
For all practical purposes, equation 2 cannot be estimated directly without
knowing the form of F. It is the distribution of ei that determines the distribution
of F. If ei is normal, F will have a cumulative normal distribution.
Following equation (2) the functional form of F is specified
with a tobit model, where ei is an independent, normally distributed error term
with mean zero and constant variance e2: It can be expressed mathematically
as:-
Yi = Xie if i* = Xie + ei >T
=0 if i* = Xie + eieT
[Equation 3]
Where Yi is the probability of adopting the alternative soil nutrient
strategy; i* is a non-observable latent variable, and T is a non-observable
threshold level. Our problem is to estimate e and e2 on the basis of the N observations
on Yi and Xi. If the non-observable latent variable i* is greater than T, the
observed qualitative variable yi that indexes adoption becomes a continuous
function of the explanatory variables, and 0 otherwise (i.e., no adoption).
The empirical model. The data requirements for this study were obtained
from both primary and secondary data sources. The primary data were collected
in Bungoma and Siaya districts of western Kenya in 1997 during a 2-month village-level
survey which involved 60 small-scale farmers.
The estimated empirical model derived from equations 1 to
3 was developed using farm variables and farmers subjective preferences for
technology-specific attributes associated with the use of alternative soil management
technologies. The dependent variable was the proportion of all available soil
nutrient replenishment technologies; use of conventional inorganic fertilisers,
use of compost manure, use of improved fallow agro forestry system, use of green
manure (mainly Tithonia diversifolia), and use of rock phosphate. The
definitions, measurements and sample characteristics of the variables are shown
in Table 1.
The technology-specific attribute A in equation 1 was specified in the empirical
model to include the following variables: YIELD, SUPPLY, VIGOUR, and CONVENIENCE,
while the farm specific factors attribute M in the same equation was specified
in the model to include; POSITION, WORKSHOP, EXTENSION, ON-FARM, and USE-LEVEL.
Table 1. Definition of variables in the empirical model
Proportion:
|
The proportion of total soil nutrient replenishment technologies that
is constituted by alternative soil nutrient replenishment technologies.
|
|
|
Independent Variables
|
|
|
|
Extension
|
Contact with extension agents, measured as a binary variable, {1} if
the farmer has been in contact with any extension agent and this influenced
his/her adoption decisions for alternative soil nutrient replenishment
technologies, {0} otherwise.
|
|
|
On-farm
|
Participation in on-farm trials, measured as a binary variable, {1} if
farmer had participated in on-farm trials, and whatever he/she saw there
influenced his/her adoption decisions for alternative soil nutrients replenishment
technologies, {0} otherwise.
|
|
|
Position
|
Leadership position of the farmer and its influence on adoption decision,
measured as a binary variable, {1} if a farmer holding a leadership position
has adopted use of alternative soil nutrients replenishment strategies,
{0} otherwise.
|
|
|
Workshop
|
Participation in agriculture oriented training workshops, {1} if farmer
attends such workshops, {0} otherwise.
|
|
|
Vigour
|
The impact of alternative soil nutrient replenishment technologies on
growth vigour of the crop in early stages of growth. This was measured
as a binary variable; {1} if the farmers perception of the impact
of alternative technologies influenced his/her adoption decisions, {0}
otherwise.
|
|
|
Convenience
|
Labour requirements and convenience in use of alternative soil nutrient
replenishment technologies and its impact on adoption decisions, measured
as a binary variable, {1} if the farmers perception of labour requirement
and convenience in use of alternative soil nutrients replenishment technologies
had an influence on his adoption decisions, {0} otherwise
|
|
|
Yield
|
Impact of a given soil nutrient replenishment strategy on yield, measured
as a binary variable, {1} if farmers perception of impact of alternative
soil nutrient replenishment strategy on yield influenced his/her adoption
decision, {0} otherwise.
|
|
|
Supply
|
adoption decisions, {0} otherwise.
|
|
|
Use-level
|
Change in the use level of conventional inorganic fertilisers following
fertiliser market liberalisation, for those farmers using such inputs,
measured as a binary input, {1} if the farmer who reduced his/ her use
of such inputs use alternative soil nutrient technologies, {0} otherwise.
|
Results and Discussion
Before presenting results of the estimated models, some
background information on the area of study about it is worth noting. A majority
of the farmers in the survey area apply organic nutrient inputs to soil (91.7%).
Farmyard manure was the most preferred organic resource having been adopted
by 72% of farmers. This was closely followed by green manure in the form of
Tithonia diversifolia (52.7%), improved fallow agroforestry systems (47%),
and phosphate rock (9.1%). Although a high number of farmers appear to have
embraced the use of alternative soil nutrient sources, the application rates
for these technologies in the study area fall below what is recommended. The
survey results show that only 52% of the farmers using Tithonia apply
1 ton and above of dry matter of the input per ha as opposed to the recommended
rate of 5 t ha-1. The remaining 48% apply less than 1 t ha-1 . For those farmers
using farmyard manure, 44% apply 1.25 t ha-1 and above opposed to the recommended
5 t ha-1. The remaining 56% apply less than 1.25 t ha-1 of the input per ha.
In the case of Minjingu phosphate rock, all the farmers using the technology
reported an application rate of 0.125 t ha-1 (= 16 kg P ha-1). This is half
the suggested rate of 0.25 t ha-1 for soil phosphorus recapitalisation with
Minjingu phosphate rock (Woomer et al., 1997).
Despite the low extension to farmer ratio in the study area,
the majority of the farmers have access to extension services (80%), an indication
of active frontline extension staff. About 71% of the survey farmers reported
that the information they receive from extension agents contains messages on
soil nutrient management. Also, 20.7% of the survey farmers attributed their
decision to adopt technologies to extension information. Of those farmers receiving
agricultural extension, 21.7% have contact with extension agents more than
five times per year, 33.3% four times per year, 28.3% three times per year,
while the remainder have contact with extension agents less than three times
per year.
A majority of the farmers who participated in this survey
(90%) participate in activities such as agricultural field days and conduct
on-farm trials. About 88.5% of such farmers reported that what they see during
field days or on-farm trials actually influences their adoption decisions. About
80% of those who attend field days adopt what they see on such occasions, 14.8%
tried on smallscale, while 5.8% only considered adopting.
From the farmers perspective, most of the organic resources
in the study area are readily accessible. For instance, 62.5% of farmers using
farmyard manure reported a reliable source. A majority (86%) of those using
Tithonia diversiflia as green manure likewise reported sufficient access.
All the farmers employing improved fallow agroforestry reported favourable supply
of seed. However, only 20% of the farmers using phosphate rock reported a reliable
supply source.
Food security is the major determining factor in farmers
decisions on whether to invest in nutrient inputs or not. A majority of the
farmers (78%) use nutrient inputs with the sole aim of improving yields and
31% of these farmers reported an improvement in yield with alternative nutrient
replenishment technologies. Food security rather than profitability was the
main factor for decisions regarding investment in farm inputs.
Farm characteristics and adoption decisions. A tobit analysis revealed
several farm variables to be positively related to adoption decisions (Table
2). The farmers participation in field-days and on-farm trials was significant(P<
0.05) in explaining the farmers subjective perceptions of the technologies
being demonstrated and the subsequent adoption decisions.
Table 2. Estimated tobit model results for adoption using only
farm and farmer related variables (Maximum Likelihood Estimates)
Independent Variables
|
Ba
|
P-values
|
t-values
|
Exp (B)b
|
|
|
|
|
|
On-farm
|
0.121
|
0.053
|
2.280*
|
0.105
|
Workshop
|
0.215
|
0.051
|
4.228**
|
0.138
|
Extension
|
0.954
|
0.055
|
1.728*
|
0.630
|
Position
|
0.506
|
0.070
|
7.210
|
0.519
|
Use-level
|
0.166
|
0.066
|
2.524**
|
0.175
|
Log-likelihood function =-14.802
**, Significant at 1%
*, Significant at 5%.
aB=coefficient
bExp (B) = product of equation 3
Conducting on-farm trials and participation in field demonstrations
using alternative technologies exposes farmers to the benefits associated with
the use of such technologies. This definitely has a positive influence on the
way such farmers perceive the new technologies and hence its significance in
influencing their adoption behavior. These results agree with what has been
reported by Hussain et al. (1994) in Pakistan. Dorfman (1996) working
in the USA found that a greater number of hours worked off-farm by the farmer
lowers the probability of adoption of new technologies. The results of Dorfman
(1996) agree with the findings of our study, which showed that farmers who devote
maximum attention to farming through field-days and involvement in on-farm
trials are more likely to be influenced by new technologies. The results differ
with the findings reported from Sierra Leone where none of the farm characteristics
had any significant influence on the farmers perceptions and adoption of new
rice varieties (Adesina and Zinnah, 1993).
Farmers attendance in workshops and seminars where new
technologies are discussed was significant (P<0.01) in explaining the farmers
perceptions of technologies and subsequent adoption behaviour. Despite the
importance of such activities, participation by farmers was low as 12.2% had
attended only once, 39% twice, 24.4% thrice and 24.4% more than three times.
This shows that either such workshops are seldom organised or if organised on
a regular basis, participation of farmers is not very high. However, these results
concur with the findings of Adesina and Baidu-Forson (1995) in Burkina
Faso and Guinea, where they found out that the number of times a farmer participated
in training activities influenced their perceptions and adoption of a new sorghum
variety. The implication of these results is that involvement by a majority
of the farmers in workshops will have a positive influence on farmers perceptions
and adoption of alternative soil nutrient technologies.
The results of tobit regression show contact with extension
workers to be statistically significant in explaining the farmers perception
of new technologies and their subsequent adoption behaviour. These results can
be explained from innovation diffusion theory, which states that contact with
extension agents have a positive effect on adoption (Voh, 1982; Kabede et
al., 1990; Polson and Spencer, 1991).
However, the notion that contact with extension agents per
se will influence farmers perceptions of technologies seems to be misplaced
(Hussain et al., 1994). It is the quality of information being conveyed
by extension agents and the intensity of extension effort that are key variables
in influencing adoption decisions.
Leadership position in the society was found not to be significant
in influencing farmers perceptions and adoption decisions. These results deviate
from expectations of innovation diffusion theory (Voh, 1982; Kabede et al.,
1990; Polson and Spencer, 1991). However, these findings agree with those of
Adesina and Baidu-Forson (1995) in West Africa. From an economic and social
perspective, these results can be explained by the fact that organic inputs
are considered second best alternatives. Those in leadership positions also
tend to be financially secure and would rather continue using mineral fertilisers
instead of bulky organic inputs. Farmers in leadership positions have other
commitments, and as such, do not devote maximum attention to farming. This may
have an influence on their perceptions of new technologies and hence adoption
decisions (Dorfmann,1996).
Farmers decision to reduce application rate of mineral
fertilisers was significant in explaining their perceptions and adoption of
alternative technologies. The results can be explained by factor substitution
theory, as the farmers consider the two-soil fertility enhancing inputs to be
substitutes. The farmer will substitute the less expensive organic resource
for inorganic sources as a result of change in the relative price of the latter.
Farmers perceptions of technologies and their influence on adoption.
This aspect of the study set out to determine the influence of technology-specific
attributes on farmers adoption decisions. A tobit analysis revealed all
the attributes under study to be positively related to the probability of adoption.
Reliability in supply and availability of alternative resources significantly
explained farmers adoption decisions (Table 3). These results agree with
the findings of Shakya and Flinn (1985) in eastern Tarai Province of Nepal,
Kabede et al. (1990) in Ethiopia and Adesina and Zinnah (1993) working
in West Africa.
Table 3. Estimated tobit model results for farmer adoption using
only technology-specific attributes, Western Kenya (Maximum Likelihood Estimates)
Independent variables
|
Ba
|
P-values
|
t-values
|
Exp (B)b
|
|
|
|
|
|
Supply
|
0.697
|
0.106
|
0.058*
|
0.666
|
Yield
|
0.106
|
0.105
|
1.010*
|
0.116
|
Convenience
|
0.006
|
0.103
|
6.603
|
0.035
|
Log-likelihood function = -15.46654
*, Significant at 5%.
aB=coefficient
bExp (B) = product of equation 3
The results imply that for adoption of alternative technologies
to be enhanced, the supply of such technologies should be made more reliable.
Strategies to ensure achievement of such an objective include planting of Tithonia
diversifolia on farm hedges to supplement supplies from public areas, bulking
planting seed for agroforestry species used in improved fallow systems and
appointment of stockists in strategic places for retailing of Minjingu rock
phosphate.
Influence of technology on crop yields was significant in
explaining farmers perceptions of soil nutrient technologies. Agriculture in
the area of study is both a source of food and income, and as such the higher
the yield the better the food security situation for the farmer, and the higher
the chances of having surplus for sale to earn income. A rational farmer is
likely to adopt technologies, which promise economic returns through improved
yields.
Farmers perceptions of convenience associated with the
use of alternative technologies, and labour requirements for the use of the
same technologies were not significant in explaining farmers adoption behaviour.
This outcome is partly derived from the high population density resulting in
surplus labour. This implies that doubts concerning acceptability of rock phosphate
in its dusty form because of inconvenience involved in its use should be discarded.
Vigour in plant growth was statistically significant in explaining the farmers
perceptions of technology adoption decisions. This outcome can be explained
from both physiological and psychological point of view. From a physiological
point of view, a healthy crop can survive stress conditions such as pest and
disease attack, and drought better than a crop with poor vigour. From a psychological
perspective, farmers derive pleasure from successful husbandry practices. The
implication is that the specific technologies that have proved effective in
supporting healthy growth should always be included in the cocktail
of technologies that are recommended to farmers.
Conclusions
The study has demonstrated that farm variables significantly
influence the farmers perceptions of new technologies and hence their adoption
decisions. The findings of the study have docu-mented that technology-specific
attributes inherent in new technologies condition the farmers subjective preferences
for such technologies and thus, probability of adoption.
The results of this study indicate that though some of the farmers in the region
may have adopted the technologies, the use level for such technologies is still
sub-optimal relative to the recommended rates. This has resulted in constrained
impact of the technologies on the welfare of the target group. Finally, the
current study only investigated factors that influence adoption at a single
point in time. The study also did not go further to investigate the influence
of the identified variables on intensity of adoption. We recommend that future
studies should consider the influence of identified variables on adoption over
time.
Acknowledgement
We acknowledge the financial support from Rockefeller Foundation
Forum on Agricultural Resource Husbandry. We also acknowledge financial support
from Farm Level Applied Research Management for East and Southern Africa (FARMESA).
The study received technical support from scientists and farmers working with
the ICRAF field station at Maseno - Kenya.
References
Adesina, A.A. and Baidu-Forson, J. 1995. Farmers perceptions and adoption
of new agricultural technology: Evidence from analysis in Burkina Faso and
Guinea, West Africa. Agricultural Economics 13:1-9.
Adesina, A.A. and Zinnah, M.M. 1993. Technology characteristics, farmers
perceptions, and adoption decisions: A tobit model application in Sierra Leone.
Agricultural Economics 9:297-311.
Agrarwal, B. 1983. Diffusion of rural innovations: Some analytical issues
and the case of wood-burning stoves. World Devevelopment 11:359-376
Aikens, M.T., Haven, A.Z. and Flinn, W.L. 1975. The Adoption of Innovations:
The Neglected Role of Institutional Constraints. Mimeograph, Department
of Rural Sociology, Ohio State University. Columbus, OH.
Asby, J.A. and Sperling, L. 1992. Institutionalizing participatory client
driven research and technology development in agriculture. In: Proceedings
of at the meeting of the CGIAR Social Scientists. 15-22 September, The
Hague, The Netherlands, pp.115-122.
Asby, J.A., Quiros, C.A. and Rivers, Y.M. 1984. Farmer participation in technology
development: Work with crop varieties. In: Farmer First. Farmer Innovation
and Agricultural Research. Chambers, R., Pacey, A. and Thrupp, L.A. (Eds.),
Intermediate Technology Publication, London, UK.
Benor, D., Harison, J.Q. and Barter, M.1984. Agricultural Extension:
The Training and Visit System. World Bank, Washington DC. 5:6.
Chapman, N. 1988. The Impact of T & V Extension in Somalia. In: Training
and Visit Extension in Practice. Occassional Paper 8, Agricultural Administration
Unit, Overseas Development Institute, London. Howel, J. (Ed.).
Dejene, A. 1989. The training and visit agricultural extension in rain-fed
agriculture: Lessons from Ethiopia. World Development 17:1647-1659.
Dorfman, J.H. 1996. Modeling multiple adoption decisions in a joint framework.
American Journal of Agricultural Economics 78:547-557.
Feder, G., Lau, L.J. and Slade, R.H. 1985. The impact of agricultural extension:
A case study of training and visit System in Haryana, India. Staff Work. Pap.
756, World Bank, Washington DC.
Feder, G., Slade, R.H. and Sundaram, A.K. 1986. The training and visit extension
system: An analysis of operations and effects. Agricultural Administration
2: 33-59.
Feder, G. and Slade, R. 1986. The impact of agricultural extension: The training
and visit system in India. World Bank Research Observer 1:139-161.
Green, D.A.G. and Ngongola, D.H. 1993. Factors affecting fertilizer
adoption in less developed countries: An application of multivariate logistic
analysis in Malawi. Journal of Agricultural Economics 44: 99-109.
Gould, B.W., Saupe, W.E. and Klemme, R.M. 1989. Conservation tillage: The
role of farm and operator characteristics and perception of erosion. Land
Economics 65:165-182.
Harper, J.K., Rister, M.E., Mjelde, J.W., Drees, B.M. and Way, M.O. 1990.
Factors influencing adoption of insect management technology. American
Journal of Agricultural Economics 72:997-1005.
Havens, A.E. and Flinn, W.L. 1976. Green revolution technology and community
development: The limits of action programs. Economic Development and Cultural
Change 23:469-481.
Hoestra, D. and Corbett, J.D. 1995. Sustainable Agricultural Growth for
the Highlands of East and Central Africa. Prospects 2020. International
Food Policy Research Institute, Washington, DC.
Hussain, S.S., Byerlee, D. and Heisey, P.W. 1994. Impacts of training and
visit extension system on farmers knowledge and adoption of technology:
Evidence from Pakistan. Agricultural Economics 10:39-47.
Just, R. and Zilberman, D. 1983. Stochastic structures, farm size, and technology
adoption in developing agriculture. Oxford Economics Papers 35: 307-328.
Kabede, Y., Gunjal, K. and Coffin, G. 1990. Adoption of new technologies
in Ethiopian agriculture: The case of Tegulet-Bulga District, Shoa Province.
Agricultural Economics 4: 27-43.
Kivlin, J.E. and Fliegel, F.C. 1966a. Farmers perceptions of farm practice
attributes. Rural Sociology 31:197-201.
Kivlin, J.E. and Fliegel, F.C. 1966b. Attributes of innovations as factors
in diffusion. American Journal of Sociology 72:235-248.
Kivlin, J.E. and Fliegel, F.C. 1967. Differential perceptions of innovations
and rate of adoption. Rural Sociology 31:197-206.
Lynne, G.D., Shonkwiler, J.S. and Rola, L.R. 1988. Attitudes and farmer conservation
behaviour. American Journal of Agricultural Economics 70:12-19.
Moore, M. 1984. Institutional development, The World Bank and Indias
new agricultural extension programme. Journal of Development Studies
20: 303-317.
Niang, A.I., Amadalo, B.A., Gathumbi, S.M., Otieno, J.H.O., Obonyo,
C.O. and Obonyo, E. 1996. KEFRI/KARI/ICRAF AFRENA, Maseno Project.
AFRENA Report No. 10. ICRAF, Nairobi.
Nekesa, P., Maritim, H.K., Okalebo, J.R. and Woomer, P.L. 1999. Economic
analysis of maize-bean production using a soil fertility replenishment product
(PREP-PAC) in western Kenya. African Crop Science Journal 7:585-590.
Norris, P.E. and Batie, S.S. 1987. Virginia farmers soil conservation decisions:
An application of Tobit analysis. Southern Journal of Agricultural Economics
19:79-89.
Nowak, P.J. 1987. The adoption of agricultural conservation technologies:
Economic and diffusion explanations. Rural Sociology 52:208-220.
Omare, M.N. 1998. Factors influencing use of fertilizer in Vihiga District
and Farmers Perceptions of PREP-PAC. M.Phil Thesis, Moi University,
Kenya.
Pitt, M.M. and Sumodiningat, G. 1991. Risk, schooling, and the choice of
seed technology in developing countries: A meta-profit function approach.
International Economics Review 32:457-473.
Polson, R.A. and Spencer, D.S.C. 1991. The technology adoption process in
subsistence agriculture: The case of cassava in South Western Nigeria. Agricultural
Sytems 36:65-77.
Reijntjes, C., Haverkrot, B. and Waters-Bayer, A. 1992. Farming for the
Future. An Introduction to Low External Input and Sustainable Agriculture.
Macmillan Press, London.
Rogers, E.M. 1962. Diffusion of innovations. Free Press, Glancoe,
IL.
Roling, N. 1988. Extension Science: Information Systems in Agricultural
Development. Wye Studies in Agricultural and Rural Development. Cambridge
University Press, UK.
Shakya, P.B. and Flinn, J.C. 1985. Adoption of modern varieties and fertilizer
use on rice in Eastern Tarai of Nepalese. Journal of Agricultural Economics
36:409-419.
Strauss, J., Babosa, M., Texeira, S., Thomas, D. and Gomes, R. 1991. Role
of education in adoption of technology: A study of upland rice and soy bean
farmers in Central-West Brazil. Agricultural Economics 5:341-359.
Voh, J.P. 1982. An Exploratory Study of Factors Associated with Adoption
of Recommended Farm Practices among Giwa Farmers. Samara Miscellaneous
Paper 73. Institute for Agricultural Research, Ahmadu Bello University, Zaria
Nigeria.
Woomer, P.L., Bekunda, M.A., Karanja, N.K., Moorehouse, T. and Okalebo, J.R.
1998. Agricultural resource management by smallhold farmers in East Africa.
Nature and Resources 34:22-33.
Woomer, P.L., Okalebo, J.R. and Sanchez, P.A. 1997. Phosphorus replenishment
in western Kenya: from field experiments to an operational strategy. African
Crop Science Conference Proceedings 3:559-570.
Yapa, L.S. and Mayfield, R.C. 1978. Non adoption of innovation: Evidence
from discriminant analysis. Economic Geography 54:145-156.
©1999, African Crop Science Society
|