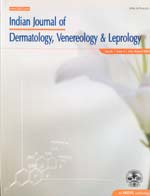
|
Indian Journal of Dermatology, Venereology and Leprology
Medknow Publications on behalf of The Indian Association of Dermatologists, Venereologists and Leprologists (IADVL)
ISSN: 0378-6323 EISSN: 0973-3922
Vol. 71, Num. 2, 2005, pp. 106-108
|
Indian Journal of Dermatology, Venereology, Leprology, Vol. 71, No. 2, March-April, 2005, pp. 106-108
Studies
Neural network algorithm to predict severity in epidermolysis bullosa simplex
Eapen BellRaj
Department of Dermatology, Atlas Star Medical Centre, Dubai
Correspondence Address:Atlas Star Medical Centre, P.O. Box - 112392, Dubai
beapen@emirates.net.ae
Code Number: dv05033
ABSTRACT
BACKGROUND AND AIMS: There are various genotypic variations
known for the four phenotypic presentations of epidermolysis bullosa
simplex (EBS). A neural network algorithm may be used to find the relationship
between the various factors responsible for a particular phenotypic presentation.
We assessed the value of neural network to predict the prognosis of epidermolysis
bullosa simplex.
METHODS: Cases of EBS in which sequencing had been performed to
identify the position and type of mutation were collected by literature
search and the resulting data was analyzed using neural network algorithm.
RESULTS: The statistical prediction had an accuracy rate of 78%.
CONCLUSION: Neural networks can identify hidden patterns in a
huge database without the intervention of a skilled statistician. It has
the potential to change the way we analyze clinical and experimental data
at present.
INTRODUCTION
Epidermolysis bullosa simplex (EBS) is a genetic disease with predominantly autosomal dominant inheritance, characterized by fragility of the skin caused by minor trauma.[1] Severity
of disease in the four clinical sub-types of EBS ranges from relatively
mild blistering of the hands and feet in the Weber Cockayne (EBS-WC)
type to more generalized blistering in the Koebner′s type (EBS-K),
EBS with mottled pigmentation (EBS-MP), and the Dowling Meara variety
(EBS-DM) which can even be fatal.[2]
All four forms of EBS are caused by mutation in either the Keratin 5
gene (KRT5, gi 27503816) or the Keratin 14 gene (KRT14 gi 27769301).[3] KRT5 and KRT14 have 590 and 472 amino acids respectively and they form obligatory heterodimers, which self-assemble into 10 nm intermediate filaments (IF). Like all IF proteins, they have a central -helical rod domain, flanked by non-helical head and tail domains. The helix initiation region, linker region and the helix termination region are the mutational hot spots.[4] Hence the clinical subtype and the severity could depend on various factors like the location of mutation, alteration in the type of amino acid, and the type of keratin involved.
Neural network is a statistical analysis tool, i.e. it lets us build behavior models starting from a collection of examples (defined by a series of numeric or textual descriptive variables) of this behavior.[5] The neural net, ignorant at the start, through a learning process, becomes a model of the dependencies between the descriptive variables and the behavior to be explained. The model is automatically and straightforwardly built from the data with no intervention needed at any stage. However, the success of neural networks largely depends on their architecture, their algorithm, and the choice of features used in training. Unfortunately, determining the architecture of a neural network is a trial-and-error process.
Several mutations have been identified in EBS following sequence analysis of 28 cases.[6] We performed neural network analysis on this data to find hidden patterns and an attempt was made to predict the phenotypic presentation based on the mutation.
METHODS
Twenty eight Cases of EBS in which sequencing had been performed to
identify the position and type of mutation were collected by a literature
search. A numeric code was assigned to the type of mutation [Table
- 1].
A neural network software called Neunet Pro marketed by Cormac Technologies
Inc, Canada was used for the analysis. A trial version of the software
is available for free download from http://www.cormactech.com/neunet/index.htm
The variables included for ′training′are explained in [Table
- 2].
Shuffling of data rows was done prior to training. Finally the ′SFAM′ method
(Simplified Fuzzy Adaptive Resonance Theory Map) of the software was
used to predict the ′disease′variable.
The first 24 rows were selected as the ′training set′.
The ′testing set′comprised
the last 9 rows which included 4 rows that were not exposed during training.
RESULTS
The details of the ′training set′ and ′testing set′of
patients are tabulated in [Table
- 3].
DISCUSSION
Transmission electron microscope examination of a skin biopsy or immunofluorescent
antibody/antigen mapping is the sine qua non for the diagnosis of EBS
at present.[8] The severity
of the disease ranges from relatively innocuous EBS-WC to potentially
fatal EBS-DM. Though prenatal diagnosis is possible with the latest molecular
testing techniques, predicting the severity of the disease is difficult
as there are many genotypic variations known for each phenotypic presentation.
This new statistical method may be of use in this regard.
Neural network algorithm has been successfully employed for predicting
prostatic cancer[9] and for
other applications in dermatology like skin capillary network recognition.[10] However
in our case, there is only a limited amount of data available and the
accuracy rate of the prediction is only 78%. Hence the utility
of this prediction algorithm is questionable. However, this highlights
the worth of ′neural networks′as
a statistical analysis tool that can be effectively used to find hidden
patterns in large databases related to dermatology.
REFERENCES
1. | Mitsuhashi Y, Hashimoto I. Genetic abnormalities and clinical classification of epidermolysis bullosa. Arch Dermatol Res 2003;295:S29-33. Back to cited text no. 1 [PUBMED] [FULLTEXT] |
2. | Sorensen CB, Andresen BS, Jensen UB, Jensen TG, Jensen PK, Gregersen N, et al. Functional testing of keratin 14 mutant proteins associated with the three major subtypes of epidermolysis bullosa simplex. Exp Dermatol 2003;12:472-9. Back to cited text no. 2 |
3. | Leigh IM, Lane EB. Mutations in the genes for epidermal keratins in epidermolysis bullosa and epidermolytic hyperkeratosis. Arch Dermatol 1993;129:1571-7. Back to cited text no. 3 [PUBMED] |
4. | Wlison KW, Coulombe PA, Fuchs E. The roles of K5 anf K14 Head, Tail, and R/KLLEGE domains in Keratin filament assembly in vitro. The J Cell Biol 1992;119:401-14. Back to cited text no. 4 |
5. | Xu ZB, Qiao H, Peng J, Zhang B. A comparative study of two modeling approaches in neural networks. Neural Netw 2004;17:73-85. Back to cited text no. 5 [PUBMED] [FULLTEXT] |
6. | Wojnarowska F, Eady RA, Burge SM. Bullous Eruptions In: Champion RH, Burton JL, Burns DA, Breathnach SM, editors. Rook Textbook of Dermatology. 6th Ed. Blackwell science; 1998. p. 1819-21. Back to cited text no. 6 |
7. | Moog U, de Die-Smulders CE, Scheffer H, van der Vlies P, Henquet CJ, Jonkman MF. Epidermolysis bullosa simplex with mottled pigmentation: Clinical aspects and confirmation of the P24L mutation in the KRT5 gene in further patients. Am J Med Genet 1999;86:376-9. Back to cited text no. 7 [PUBMED] [FULLTEXT] |
8. | Petronius D, Bergman R, Ben Izhak O, Leiba R, Sprecher E. A comparative study of immunohistochemistry and electron microscopy used in the diagnosis of epidermolysis bullosa. Am J Dermatopathol 2003;25:198-203. Back to cited text no. 8 [PUBMED] [FULLTEXT] |
9. | Babaian RJ, Fritsche H, Ayala A, Bhadkamkar V, Johnston DA, Naccarato W, et al. Performance of a neural network in detecting prostate cancer in the prostate-specific antigen reflex range of 2.5 to 4.0 ng/mL. Urology 2000;56:1000-6. Back to cited text no. 9 |
10. | Sainthillier JM, Gharbi T, Muret P, Humbert P. Skin capillary network recognition and analysis by means of neural algorithms. Skin Res Technol 2005;11:9-16. Back to cited text no. 10 [PUBMED] |
Copyright 2005 - Indian Journal of Dermatology, Venereology, Leprology
The following images related to this document are available:
Photo images
[dv05033t2.jpg]
[dv05033t1.jpg]
[dv05033t3.jpg]
|