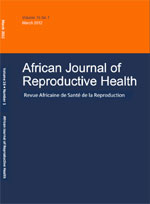
|
African Journal of Reproductive Health
Women's Health and Action Research Centre
ISSN: 1118-4841
Vol. 12, Num. 1, 2008, pp. 17-21
|
African Journal of Reproductive Health,
Vol. 12, No. 1, April
2008, pp. 17-21
Original Research Articles
Statistical Approach for Evaluation of Contraceptive Data
Approche statistique pour l’évaluation des données contraceptives
Dr. Vrijesh Tripathi, PhD (Biomedical Statistics)
Department of Mathematics & Computer Science, Faculty of Science & Agriculture University of West Indies St Augustine Campus, Trinidad & Tobago
Corresponding Address: Dr. Vrijesh Tripathi Department of Mathematics & Computer Science Faculty of Science & Agriculture University of West Indies St Augustine Campus, Trinidad & Tobago Email: vrijesh_tripathi@yahoo.com
Code Number: rh08003
ABSTRACT This article will define how best to analyse data collected from a longitudinal follow up on contraceptive use and discontinuation, with special consideration to the needs of developing countries. Accessibility and acceptability of contraceptives at the ground level remains low and it is an overlooked area of research. The author presents a set of propositions that are closer in spirit to practical recommendations than to formal theorems. We will comment specifically on issues of model validation of model through bootstrapping techniques. The paper makes a presentation of a multivariate model to assess the rate of discontinuation of contraception, while accounting for the possibility that there may be factors that influence both a couple’s choice of provider and their probability of discontinuation.
KEY WORDS: IUCD, contraception, discontinuation, logistic, Cox model
RÉSUMÉ
Cet article définira la meilleure façon d’analyser les données collectionnées à partir d’une surveillance des suites thérapeutiques sur l’utilisation et la suspension de l’utilisation du contraceptif, en considérant surtout les besoins des pays en voie de développement. L’accès aux contraceptifs ainsi que leur acceptation à la base sont restés faibles et c’est un domaine de recherche qui est négligé. L’auteur présente une série de propositions qui rapproche plus de l’esprit aux recommandations pratiques qu’aux théorèmes formels. Nous ferons des commentaires spécifiques sur les questions de la validation du modèle. L’article présente un modèle multifactoriel qui permet d’évaluer le taux d’interruption de la contraception, tout en tenant en compte le fait qu’il peut y avoir des facteurs qui influent, à la fois, sur le choix d’un couple d’un dispensateur de soins et leur probabilité de l’interruption.
Introduction The choice of a statistical technique can be sometimes as daunting a task as collecting data. This article will define how best to analyse data collected from a longitudinal follow up survey on contraceptive use and discontinuation, with special consideration to the needs of the developing countries, where accessibility and acceptability remain two major blocks in achieving better reproductive health needs. It is important to remember that funding is often available for controlled clinical trials which address the medical rather than social determinants of contraceptive accessibility and acceptability. Also, that the contraceptive needs of populations of developing country may vary greatly from the needs of couples in developed nations, where access to education and quality of health care is better. There are gaps in statistical understanding of assessing early discontinuation of contraceptives. Apart from method failure and loss to follow-up, which remain a statistical problem in clinical trial data, social determinants such as religion, age, distance from the nearest health centre, education, social taboos and traditional beliefs need to be focused upon. To take the example of IUCD, the review of literature reveals that the discontinuation rate of IUCD at the end of one year are around 10 percent and could even reach as high as 40 percent in developing countries. However, most of these studies focus on dynamics of continuation rather than discontinuation often combining expulsion and failure with other motivational and medical side effects (Salhan & Tripathi, 2004; Tripathi et al, 2005; Saxena, 1996 & Cotton N et al., 1992). This paper is meant to give direction to researchers who wish to focus upon the dynamics of discontinuation of contraceptives in developing countries. Frequently, allocation of funds are made available for increasing contraceptive use and informed choices without focusing upon the needs and factors of early discontinuation of contraceptives. It needs to be emphasized that such studies in developing countries are of import for determining social acceptability of reproductive choices of population.
The review of literature is an important tool to identify the number of factors associated with discontinuation of contraceptive. However, it is helpful to classify the same under categories of socio-economic and demographic determinants, reproductive history, counselling and quality of care factors and medical side-effects. Though the medical factors override all other determinants due to their urgency, other factors are important from the point of view of policy formulation and its execution. Moreover, as can be hypothesized, factors associated with early discontinuation within six months will be quite different from those factors beyond one year.
It is imperative to remember that the discontinuation pattern of contraceptive use is complex (Phillips, 1991 and Trussel, 1991). This complexity is due to the fact that discontinuation of methods is often high in early months of use, declines with time, and stabilizes as users who continue through the early period of use are comprised of women who are progressively tolerant of method as time progresses. The underlying relationship of discontinuation with time may co-vary with posited determinants. The study of contraceptive effectiveness has been largely governed by Potter and Tietze and Lewitt who had specified procedures for studying levels of use-effectiveness and estimating of component decremental factors that explained termination (Phillips, 1991). But, traditional Life table techniques have been replaced with multiple regression techniques that utilize the maximum information available through data. For studies with staggered entry times and with a maximum of cases not experiencing the event of interest, any model that does not utilize all the information is not able to provide a powerful assessment of the relationship between risk factors and the event of interest (Katz,1999).
The best suited framework for analysing discontinuation is a community based longitudinal survey. Survival Analysis makes use of the contribution of censored cases and is therefore, more useful for analysing discontinuation in contraceptive data.
Comparison between Logistic and Cox regression analysis- In analyzing data on survival, one possibility is to classify individuals according to their status at a fixed duration of follow-up. Thus two groups are created: those who continue and those who do not. Logistic regression can be used to analyze the data from these two groups with the objective of estimating the probability of continuation given the length of time. Logistic regression coefficients can be computed to describe the relationship between the independent variables and log odds of discontinuation. When a linear regression coefficient is estimated for a variable, the implication is that a unit change in the value should always have the same effect on the output. This is the case with independent binary variables but with ranked variables several gradients may occur as the variable moves through its series of ordinal categories. A common choice to overcome the linearity assumption is to expand the variable to a higher power before adding it to the model. The linearity assumption should be checked irrespective of the use of statistical techniques while the proportionality assumption is checked only in the case of Cox Proportional Hazard analysis.
The Cox model is able to adjust simultaneously for the influence of several concomitant variables. This property of the model makes it superior to the earlier methods such as log-rank test, used to compare the survival in subgroups of patients. The Cox model has somewhat stronger assumptions of the covariates having simultaneously a proportional effect on the hazard function. The assumption is that the hazards for persons with different patterns of covariates are constant over time. If the risk of outcome associated with a particular variable is higher at one point in time and lower at another, a single coefficient cannot represent that relationship. In literature, there are three approaches for evaluating the proportionality assumption of the Cox model namely, a graphical procedure, a goodness of fit testing procedure, and a procedure that involves the use of time dependent variable. Each of these procedures has some merits and demerits. After an in depth understanding of each of these methods, Kleinbaum (199 6) has recommended that at least two of these methods should be employed.
Several statisticians (Ingram and Kleinman1, 989 and Green and Symons, 1983) have examined the relationship between the results obtained from logistic regression analysis and those from the Cox model. Ingram and Kleinman assume that the true population distribution follows a Weibull distribution with one independent variable that took on two values, and that the distribution could be thought of as group membership. Through Monte Carlo simulation, the authors concluded that the estimated regression coefficients were similar (same sign and magnitude) for logistic regression and Cox model when the patients were followed for a short time. They classified the cases as alive if they survived the follow-up period. They noted that for a short period, relatively few patients die. The range of survival times for those who die would be less than for a longer period. But, as the length of follow-up increased, the logistic regression coefficients increased in magnitude but those for the Cox model stayed the same. The estimates of the standard error for the Cox model decreased as the follow-up time increased. Secondly, the logistic regression coefficient became very biased when there was greater censoring in one group than in the other, but the regression coefficients from the Cox model remained unbiased (50% censoring was used). Third, when the proportion dying is small, the estimate regression coefficients were similar for the two methods. As the proportion dying increased, the regression coefficient for the Cox model stayed the same and their standard errors decreased. The logistic regression coefficient increased as the proportion dying increased. And lastly, that minor violation of the proportional hazards assumption had a small effect on the estimates of the coefficients for the Cox model.
But in the case of contraceptives, more people are likely to discontinue within a short period of time post-enrollment. It is essential therefore, that biases inherent in the logistic model should be avoided given the fact that over time, the process of discontinuation stabilizes and different variables behave variably over time. Desire for another child is a covariate which would show no significant effect at 6 months yet as can be hypothesized it would play a significant role in analysis dealing with continuation rates at one year or above. This covariate also needs to be seen in correlation to a covariate intention to continue.
When time dependent variables are used to assess the proportional hazard assumption for time- independent variable the Cox model is extended to contain product (i.e interaction) terms involving the time-independent variable being assessed and some function of time. A timedependent variable is defined as any variable whose value for a given subject may differ over time. In contrast, a time-independent variable is a variable whose value for a given subject remains constant. To test the no interaction assumption, a likelihood test compares log likelihood statistics for the interaction model and the no interaction model. The correlation coefficient is a bivariate statistic that measures how strongly two variables are related to one another. To determine how correlated independent variables are a correlation coefficient matrix with all proposed independent variables may be run. Two variables correlated at >0.8 may cause multicollinearity problems in analysis. If the variables are highly related, consider omitting the variable, using an “and/ or” clause or create a scale.( Katz, 1999).
Therefore, as opposed to intervention studies, the Cox model should be preferred over other techniques for analyzing factors associated with early discontinuation of contraceptive. The Cox model is a safe choice in view of the uncertainty regarding whether or not a considered parametric model is appropriate. In addition to its robustness, the coefficient in the exponential part of the model can be estimated without specifying the baseline hazard. In the absence of the specific baseline hazards function, the hazard function and its corresponding survival curve can also be estimated for the Cox model. Thus, the primary information desired for a survival analysis, namely, a hazard ratio and a survival curve, may be obtained using a minimum of assumptions. This model is also preferred over the logistic model when survival time information is available and there is censoring (Kleinbaum, 1996 and Christensen, 1987).
Variables that have a moderate influence upon discontinuation (p<0.25) can then be taken further for multivariate analysis. The covariates identified in the final model can be validated by checking for overfitting/ under-fitting of the model. An uncritical application of modeling techniques can result in models that poorly fit the dataset at hand, or, even more likely, inaccurately predict outcomes on new subjects. One must know how to measure qualities of a model’s fit in order to avoid poorly fitted or over fitted models. Measurements of predictive accuracy can be made through an easily interpretable index of predictive discrimination and through methods for assessing calibration of predicted survival probabilities. Methods for validating models include performing a test analysis on a subsample of patients followed by a subsequent validation analysis on the remaining patients, repeating the analysis on an independent sample of patients, or using “jackknife” or “bootstrap” procedures in which the same analysis is performed multiple times on a series of subsets from the same data set to investigate the stability of coefficients and predictive ability of the model (Efron & Tibshirani, 1993).
Conclusion
Thus, it is important to carefully examine the type of data, length of follow-up, number of acceptors who discontinue or are censored at the end of follow-up period before choosing the most effective statistical model for analysis. The paper highlights the strengths of Cox model for analysis. The advantages of analysing the discontinuation patterns are immense with reference to developing countries since it would mean better facilitation and infrastructural development rather than the trend of increasing the use of contraceptives to built a society more aware of its reproductive choices.
REFERENCES
- Christensen, E. (1987). Multivariate Survival Analysis Using Cox Regression Model. Hepatology 7, 1346-1358.
-
Cotton N et al. (1992). Early discontinuation of contraceptive use in Niger and The Gambia.International Family Planning Perspectives 18(4), 145–149
-
Efron, B. and Tibshirani, R. (1993). An Introduction to Bootstrap. Chapman and Hall, New York.
-
Green, M.S and Symons, M.J. (1983. A comparison of logistic risk function and the proportional hazards model in prospective epidemiologic studies. Journal of Chronic Disease 36, 715-24.
- Ingram D.D. and Kleinman, J.C. (1989). Empirical comparison of proportional hazards and logistic regression models. Statistics in medicine 8, 525-38.
- Katz, M.H. (1999). Multivariable Analysis: A Practical Guide for Clinicians. Cambridge University Press, UK.
- Kleinbaum, D.G. (1996). Survival Analysis: A Self Learning Text. Springer, New York.
- Phillips, J.F., Mundigo, A., Chamratrithirong, A. (1991). The Correlates of Continuity in Contraceptive use. Journal of Family Welfare of India 35, 3-17.
- Salhan S, Triapthi V (2004). Factors influencing discontinuation of intrauterine contraceptive devices: an assessment in the Indian Context. The European Journal of contraceptive and Reproductive Health Care 9(4),245-59.
- Saxena, B.N. (1996). Reproductive Health in India. Advances in Contraception 12, 265-70.
- Tripathi V, Nandan D, Salhan S (2005). Determinants of Early Discontinuation of IUCD in Rural Northern District of India: A Multivariate Analysis and its Validation. Journal of Biosocial Science 37(3), 319-332.
- Trussel, J. (1991). Methodological Pitfalls in the Analysis of Contraceptive Failure. Statistics in Medicine 10, 201-220.
Copyright 2008 - Women's
Health and Action Research Centre, Benin City, Nigeria
|